Aakash Nama
Ms. Pragya Bharti
Keywords:
Diabetic Retinopathy, Retinal Fundus Images, Deep Learning, Convolutional Neural Network, STARE Database, DRIVE Database, Vessel Segmentation, Cotton Wool Spots, Preprocessing, Image Classification, Computer-Aided Diagnosis, Medical Image Analysis, Retinal Abnormalities, Severity Grading, Ophthalmology.
Abstract:
Diabetic Retinopathy (DR) is a progressive eye disease and a leading cause of vision impairment and blindness among diabetic individuals worldwide. Early diagnosis and timely intervention are essential to prevent permanent vision loss. This research presents the development of a computer-aided diagnostic (CAD) system that leverages deep learning for the automatic detection and severity analysis of diabetic retinopathy using retinal fundus images.The proposed system focuses on three primary components: retinal blood vessel segmentation, detection of cotton wool spots (white lesions indicating disease severity), and classification of retinal images into normal and abnormal categories. The methodology integrates spatially adaptive contrast enhancement with a modified Tyler Coye algorithm and Hough line transformation to improve blood vessel visibility and reconstruction. This hybrid vessel segmentation technique significantly enhances the detection of fine vascular structures and pathological features.A deep Convolutional Neural Network (CNN) model is designed and trained on publicly available STARE and DRIVE datasets. Preprocessing techniques such as normalization, data augmentation, and contrast adjustment are employed to improve the robustness and accuracy of the model. Experimental results indicate that the system achieves 82% classification accuracy on the STARE dataset and 91.5% on the DRIVE dataset with preprocessing, outperforming standard deep learning approaches like ResNet under the same conditions.This study demonstrates the effectiveness of the proposed deep learning-based CAD system in replicating ophthalmological evaluations for DR screening. The system’s scalability and high performance make it particularly valuable for early-stage diagnosis and grading of diabetic retinopathy in clinical and resource-constrained environments. Overall, the research contributes a reliable and automated solution to assist healthcare professionals in managing diabetic eye care, thereby reducing the global burden of vision loss due to diabetic retinopathy.
|
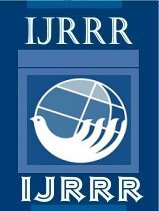
International Journal of Recent Research and Review
ISSN: 2277-8322
Vol. XVIII, Issue 1
March 2025
|
PDF View
PUBLISHED
March 2025
ISSUE
Vol. XVIII, Issue 1
SECTION
Articles
|