Richa Sharma
Ms. Pragya Bharti
Keywords:
Paddy leaf diseases, image processing, machine learning, disease classification, Support Vector Machine, enhanced SVM, precision agriculture, paddy disease detection, leaf image segmentation, agricultural automation, crop health monitoring, disease identification, paddy cultivation, digital image analysis, intelligent system.
Abstract:
This research presents the development and evaluation of an intelligent system for the classification of paddy leaf diseases using image processing and machine learning techniques. Recognizing the critical need for early detection of crop infections that threaten paddy cultivation, the system captures digital images of both healthy and diseased paddy leaves and applies pre-processing methods such as noise removal, contrast enhancement, and segmentation to isolate disease-affected regions. These refined images are then fed into various machine learning classifiers, including Random Forest, K-Nearest Neighbors, Naive Bayes, Support Vector Machine (SVM), and an enhanced SVM. The system was tested on a dataset comprising common paddy diseases—blast, bacterial blight, sheath rot, and brown spot. Performance metrics such as accuracy, precision, recall, F1-score, and false positive rate were used to assess model effectiveness. The enhanced SVM classifier achieved the highest classification accuracy of 90%, along with superior precision and recall, demonstrating its robustness in disease detection. A user-friendly graphical interface was also developed for real-time classification, supporting its practical application in agriculture. This study underscores the potential of combining image processing with optimized machine learning models for effective paddy disease management and highlights its relevance in precision agriculture to improve crop health and yield.
|
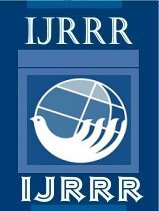
International Journal of Recent Research and Review
ISSN: 2277-8322
Vol. XVIII, Issue 1
March 2025
|
PDF View
PUBLISHED
March 2025
ISSUE
Vol. XVIII, Issue 1
SECTION
Articles
|