Sunil Kumar Saini
Ms. Pragya Bharti
Keywords:
Arrhythmia Prediction, Data Mining, Classification Algorithms, Feature Selection, Machine Learning, Electrocardiogram (ECG).
Abstract:
The state arrhythmia, a state of irregular heartbeat, is a significant health problem that can lead to serious complications including sudden cardiac arrest. The exact and initial prediction of arrhythmia is important for effective medical intervention and patient care. With progress in machine learning and data mining, the classification algorithm has become an essential tool for detecting arrhythmia from the electrocardiogram signals (ECG). However, the high height of the ECG dataset creates a challenge, making the facility's electoral technique to improve model performance, reduce calculation complexity and increase the interpretation. This article offers various data mining classification algorithms such as decision trees, Support Vector Machines (SVM), k-Nearest Neighbors (k-NN), Naïve Bayes, Random Forest, and Deep Learning models employed for arrhythmia prediction. In addition, we discover different functional choice techniques, including filters, cover and built -in methods, to identify the most relevant properties that contribute to better classification accuracy. We also discuss hybrid approaches that integrate many classify and facilitate selection strategies to achieve increased prediction benefit. In addition, this review addresses challenges such as unbalanced data sets, noise ECG signals and model lecturers, highlighting recent progress and potential future research directions. The findings from this study serve as a valuable resource for researchers and health professionals, who aim to develop a more strong and effective arrhythmia model.
|
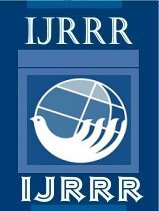
International Journal of Recent Research and Review
ISSN: 2277-8322
Vol. XVIII, Issue 1
March 2025
|
PDF View
PUBLISHED
March 2025
ISSUE
Vol. XVIII, Issue 1
SECTION
Articles
|